Welcome to Nural's newsletter where you will find a compilation of articles, news and cool companies, all focusing on how AI is being used to tackle global grand challenges.
Our aim is to make sure that you are always up to date with the most important developments in this fast-moving field.
Packed inside we have
- DeepMind pieces together ancient Greek epigrams;
- "Cultural transmission" of human skills in an AI environment;
- and, Has deep learning hit a wall ... or driven through it?
If you would like to support our continued work from £1 then click here!
Graham Lane & Marcel Hedman
Key Recent Developments
DeepMind’s new AI model helps decipher, date, and locate ancient inscriptions

What: DeepMind has created an AI model that helps restore missing text from ancient Greek inscriptions. It also suggests when the text was written and its possible geographic origins. Currently epigraphers look for textual and contextual parallels in similar inscriptions but this is difficult given the amount of existing data. The new model was trained on over 75,000 labelled Greek inscriptions.
Key Takeaways: As with many successful practical applications of AI, the new model is clearly intended as a tool to aid human experts rather than a stand-alone solution. The code is open source and available for anyone to use. The researchers emphasise that the real value of the system will be in its flexibility. Although it was trained on ancient Greek inscriptions, it could be easily configured to work with other ancient scripts such as Latin, Mayan, cuneiform or any other written manuscripts.
Blog: Predicting the past with Ithaca
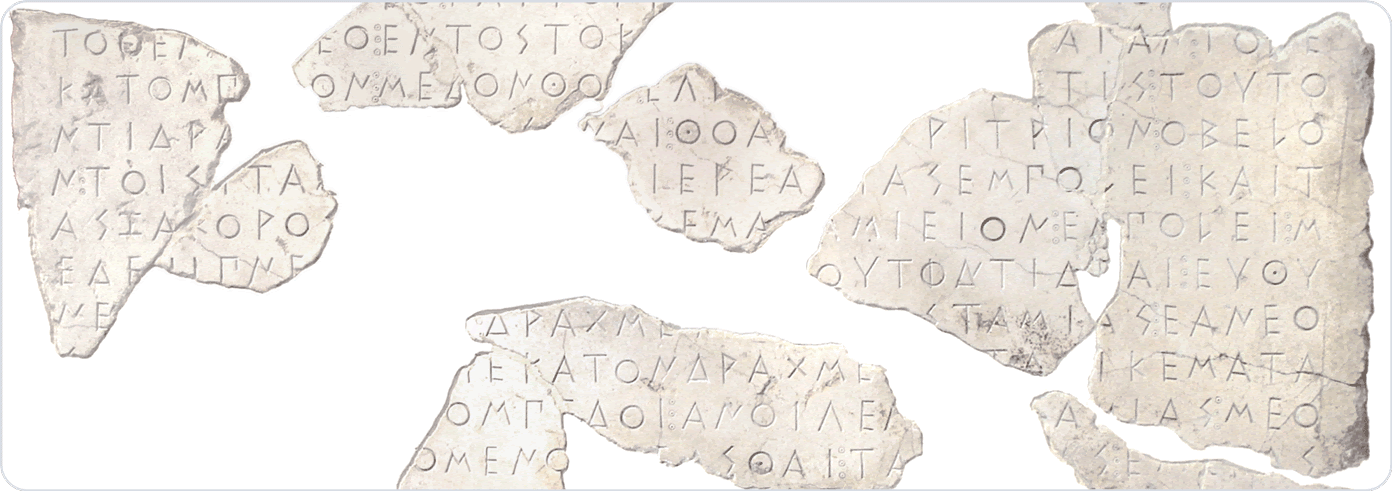
An artificial intelligence program that makes mistakes? Yes, it exists!

What: A new AI chess program does not aim to win every game but rather to predict human moves, particularly the mistakes. The objective is to be better at interacting with humans by helping humans to avoid mistakes. One application could be in healthcare where an AI system that anticipates errors could be useful in training doctors to read medical images and help them identify errors.
Key Takeaways: There is a great deal of similar work relating to the optimisation of human-AI collaboration in real world AI applications. Other recent research has investigated jointly optimising the complete human-AI system rather than the standard paradigm of simply optimising the AI model alone. Counter-intuitively, this research found that making the AI calibration less accurate (in certain circumstances) actually improved human performance.
Additionally, DeepMind has produced remarkable research demonstrating “cultural transmission” of learning from humans to an AI environment. In the research AI agents quickly learn new behaviours by observing a single human demonstration, without ever training on human data. The AI agents follow a “privileged bot” that can complete a given task correctly. Thereafter, the AI agents retain this information and can apply it to unseen, new tasks.
Deep learning is hitting a wall
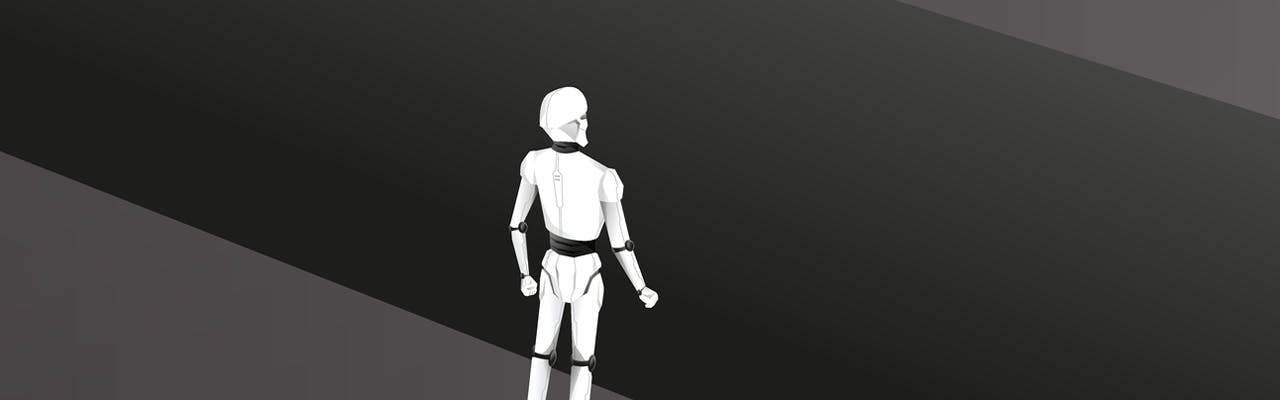
What: The article by a well-known scientist and writer offers a trenchant critique of the current state of AI stating “In time we will see that deep learning was only a tiny part of what we need to build if we’re ever going to get trustworthy AI” and that deep learning is “at its best when all we need are rough-ready results, where stakes are low and perfect results optional”. He argues that deep learning needs to be combined with human contributions in symbol-manipulating AI in order to achieve progress to generalised artificial intelligence.
Key Takeaways: This is the latest contribution to an ongoing debate, coming soon after the Chief Scientist of Facebook published a very different route to generalised artificial intelligence, based entirely on self-supervised AI systems operating with minimal help from humans. This is now also available as a detailed presentation on YouTube.
Has deep learning indeed “hit a wall”? Or has it just driven right through it?
AI Ethics
🚀 Lessons learned on language model safety and misuse
OpenAI reflect on their evolving response to misuse of GPT-3 “in the wild”; the development of novel benchmarks for language models; and the significant benefits of basic safety research for the commercial utility of AI systems.
🚀 How wrongful arrests based on AI derailed 3 men's lives
A sobering account of 3 black men in the U.S.A. wrongly identified by facial recognition software.
🚀 Maintaining fairness across distribution shift: do we have viable solutions for real-world applications?
The performance of medical applications may deteriorate when moved from one hospital to another. The conclusion of the paper is that in real-world settings this transfer is far more complex than the situations considered in current algorithmic fairness research, suggesting a need for remedies beyond purely algorithmic interventions.
Other interesting reads
🚀 This app can diagnose rare diseases from a child's face
The app is also able to cluster and differentiate rare disorders for which training data is limited or does not exist.
🚀 Geographic hints help a simple robot navigate for kilometers
A small robotic buggy with a camera, called ViKiNG, navigates distances of more than 2 kilometers across previously unseen terrain to a goal identified only by a camera photo and an approximate geographic location of the destination. The system uses two deep neural networks trained on a map and satellite images respectively (both from Google) to plot the best route.
🚀 Big Tech is spending billions on AI research. Investors should keep an eye out
The figures are huge.
Alphabet (formerly Google) R&D rose from $27 billion in 2020 to £31 billion in 2021, amounting to over 12% of revenue.
Meta (formerly Facebook) R&D rose from £18 billion in 2020 to $24 billion in 2021, which was equivalent to 21% of annual sales.
Cool companies found this week
Environment
Truecircle - a UK-based computer vision startup has raised $5.5 million in pre-seed funding to apply data-driven AI to the recycling industry with the aim of improving recycling rates and ultimately reducing the demand for virgin materials.
De-extinction
Colossal - describes itself as “the de-extinction company" and aims to bring extinct species, such as the Woolly Mammoth, back to life. The company has developed a platform combining genetic sequencing with machine learning and has secured $60 million round A funding. Here is the original Jurassic Park trailer
Speech-to-text
AssemblyAI - provides APIs for speech/audio-to-text conversion, using in-house developed AI models with added features such as an auto-chapter creation and the detection of entities such as organisations or people. The company has raised $28 million in round A funding.
And Finally ...
Would you bet $10,000 that fully autonomous cars - sit in the back and watch a video - will be commonly available by the end of 2029?
AI/ML must knows
Foundation Models - any model trained on broad data at scale that can be fine-tuned to a wide range of downstream tasks. Examples include BERT and GPT-3. (See also Transfer Learning)
Few shot learning - Supervised learning using only a small dataset to master the task.
Transfer Learning - Reusing parts or all of a model designed for one task on a new task with the aim of reducing training time and improving performance.
Generative adversarial network - Generative models that create new data instances that resemble your training data. They can be used to generate fake images.
Deep Learning - Deep learning is a form of machine learning based on artificial neural networks.
Best,
Marcel Hedman
Nural Research Founder
www.nural.cc
If this has been interesting, share it with a friend who will find it equally valuable. If you are not already a subscriber, then subscribe here.
If you are enjoying this content and would like to support the work financially then you can amend your plan here from £1/month!