When tackling any global grand challenge there are tradeoffs that arise and climate change is no different, with tradeoffs appearing time and time again. Whether that be balancing afforestation with food scarcity needs or generating economic growth at the expense of increased carbon emissions, there is always a balance to be found.
The potential impact that artificial intelligence can have to tackle climate change cannot be understated, but the question of at what cost must be evaluated. It has been found that AI systems can be huge carbon emitters and there is growing awareness of the environmental implications that come with training and deploying models. A 2019 report estimated that training a single deep learning model for NLP can emit almost five times the lifetime CO2 emissions of the average car and so there is clearly an implication to be considered.
Is there an environmental problem with AI?
The environmental problems from AI stem from the the large amounts of computational power that is needed to train them. The amount of computational power required for training state-of-the-art deep learning models increased 600,000x from 2013-2019 which has deep environmental consequences alongside increasing the barrier for individual researchers to build state of the art models[5]. However, the emissions also continue into the deployment phase where the models are ran on a range of different hardware, each requiring the model parameters to be adjusted to fit with the capability of the hardware.[6]
Furthermore, a major barrier to truly understanding the environmental impact is that most ML research papers do not report energy or carbon emissions metrics.[8] This lack of reporting reduces the ability for mitigation steps to be developed and makes it harder for incentives to be created for the development of energy efficient algorithms.
However, as greater understanding has developed there has been a gradual movement towards a branch of AI termed: Green AI[5]. This branch is referring to developing green models at all stages of the workflow: from inception to deployment. In recent times, there have been a number of different approaches that have taken ahold to bring about Green AI.
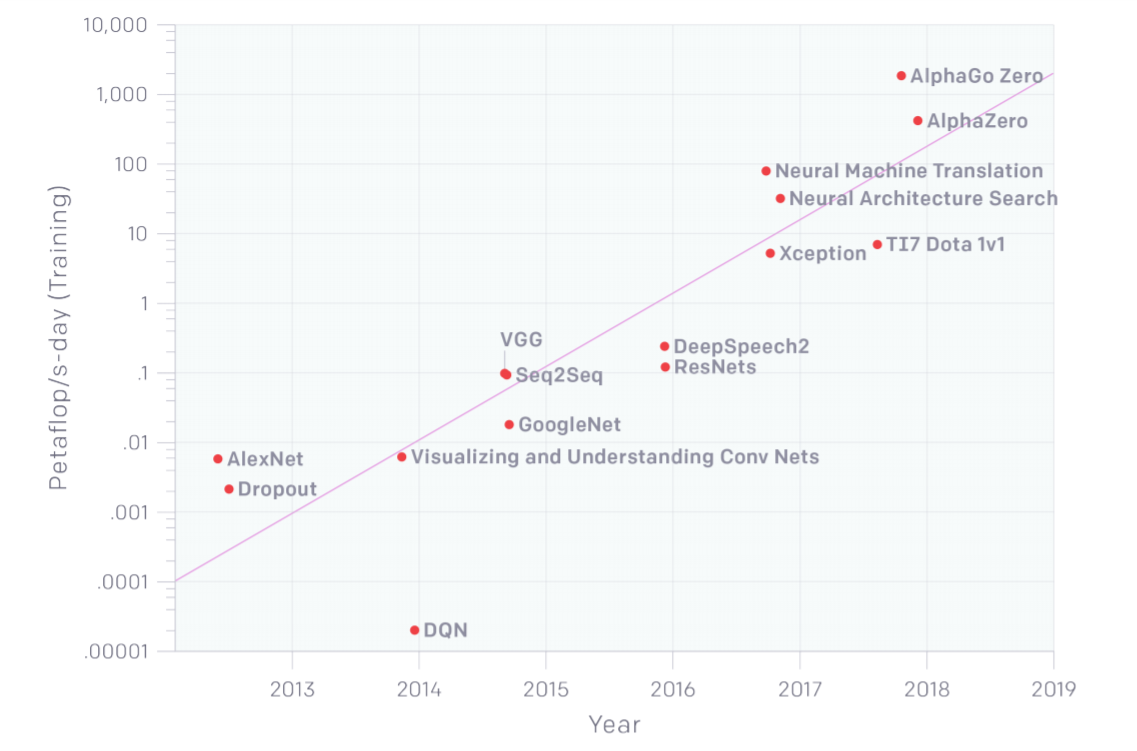
When judging deployed neural networks, its environmental impact must be a key metric.
Let's make Green AI
To make Green AI a reality, a number of different approaches are being developed and they are equally relevant for entrepreneur, regulator or researcher alike.
1 Downstream applications
Throughout this discussion, we must take into account the potential that AI has in recouping the energy consumed during its training process at a later stage as there are many cases where the opportunities unlocked are huge. One example is the startup Pachama which uses machine learning algorithms on satellite images of forests with the aim of identifying the amount of carbon captured. In doing so, they have enabled a carbon market to be created and protected over a million hectares of forest. Furthermore, both Google and Microsoft have tools which cultivate Green AI development. The former offers access to global map data alongside ML capabilities and the latter offers a combination of grants, open source tools, APIs and pre-trained models to cultivate model development for climate change.


2 Effective reporting
Effective reporting of the carbon impact of models is a basic step that will have an outsized impact. There are two sides to this coin: effective tools for tracking carbon emissions which can then lead to effective reporting.
In the field of tracking, a number of tools have sprung up. One such tool is a carbon emission tracker developed by researchers from Stanford, Facebook, Mila and McGill. The tool tracks key metrics such as python packages used and the location of training for cloud based models to evaluate how efficient a model is. The implementation of the tracker is an easy package install and they even created a leader board of energy efficient deep learning models that can be used. With effective tracking tools, reporting becomes exponentially easier and effective reporting will drive the move towards efficient algorithms being created. Effective reporting will also shift the evaluation of models away from just accuracy and towards more holistic measurements which take externalities into account.
3 Once-for-all Networks
Once-for-all (OFA) networks were developed by MIT researchers and comprise of training "one large neural network comprising many pre-trained subnetworks of different sizes that can be tailored to diverse hardware platforms without retraining.[6]" OFA networks remove the need for training a model for each new scenario or hardware type as they can search through the pre-trained models in the OFA network. This has huge implications on training speed and thus computation power needed. Check out the github to have a deeper look.
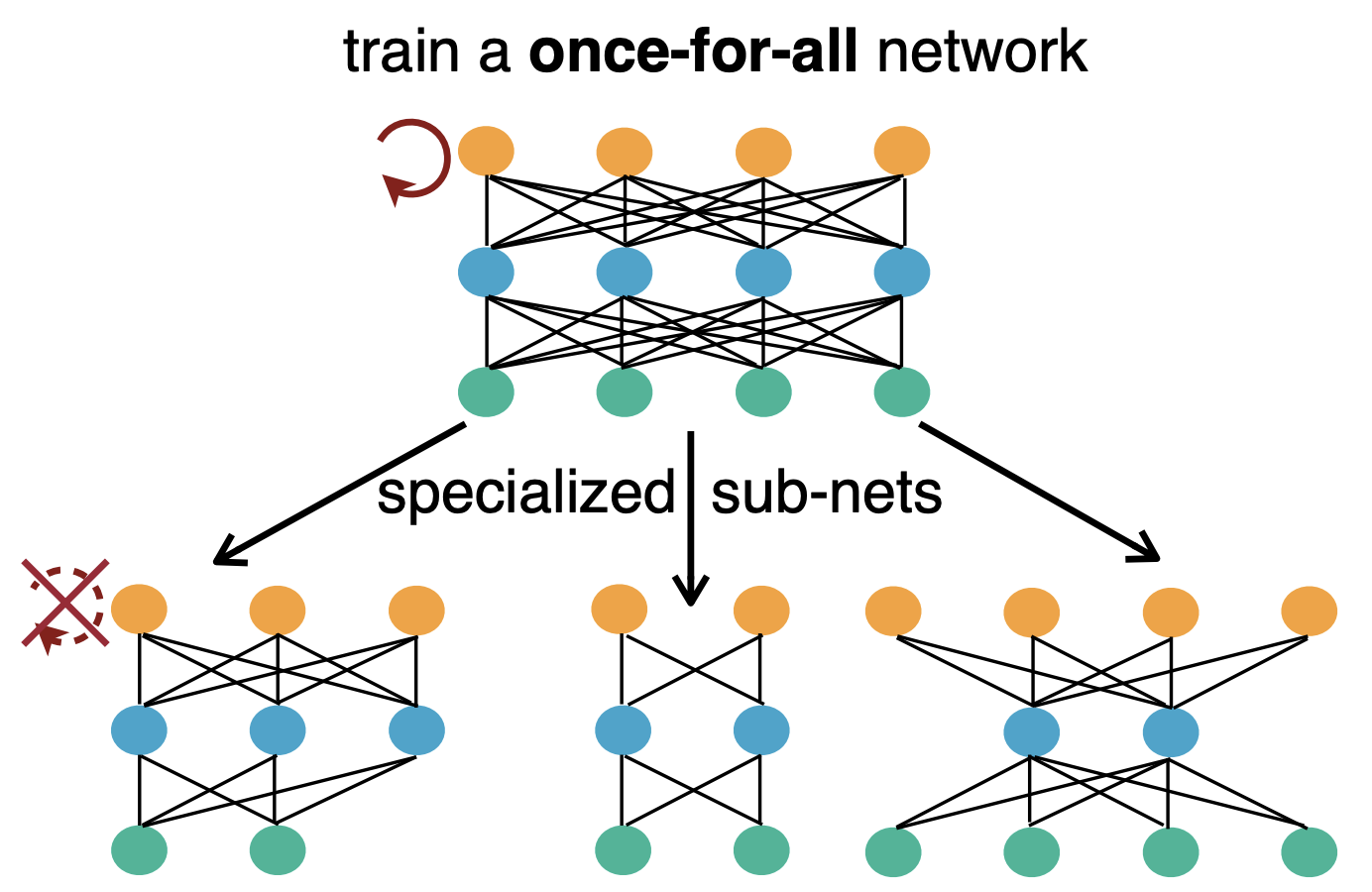
ML models have plenty of room to be optimised, not only from an accuracy standpoint but also in building Green AI that has a minimal impact on the environment.
References
[1] https://plana.earth/academy/ai-climate-change/#:~:text=AI leads to sufficient energy,can help tackle climate change
[2] https://www.oxfordfoundry.ox.ac.uk/sites/default/files/learning-guide/2019-11/New Artificial Intelligence Climate Change_ Supplementary Impact Report.pdf
[3] https://arxiv.org/pdf/1906.02243.pdf
[4] https://www.forbes.com/sites/robtoews/2020/06/17/deep-learnings-climate-change-problem/?sh=18f5ed636b43
[5] https://arxiv.org/pdf/1907.10597.pdf
[6] https://news.mit.edu/2020/artificial-intelligence-ai-carbon-footprint-0423#:~:text=MIT researchers have developed a new automated AI system for,down to low triple digits.
[7] https://www.infoworld.com/article/3568680/is-the-carbon-footprint-of-ai-too-big.html
[8] https://arxiv.org/pdf/2002.05651.pdf
[9] https://medium.com/syncedreview/one-network-to-fit-all-hardware-new-mit-automl-method-trains-14x-faster-than-sota-nas-1120c6787eb8